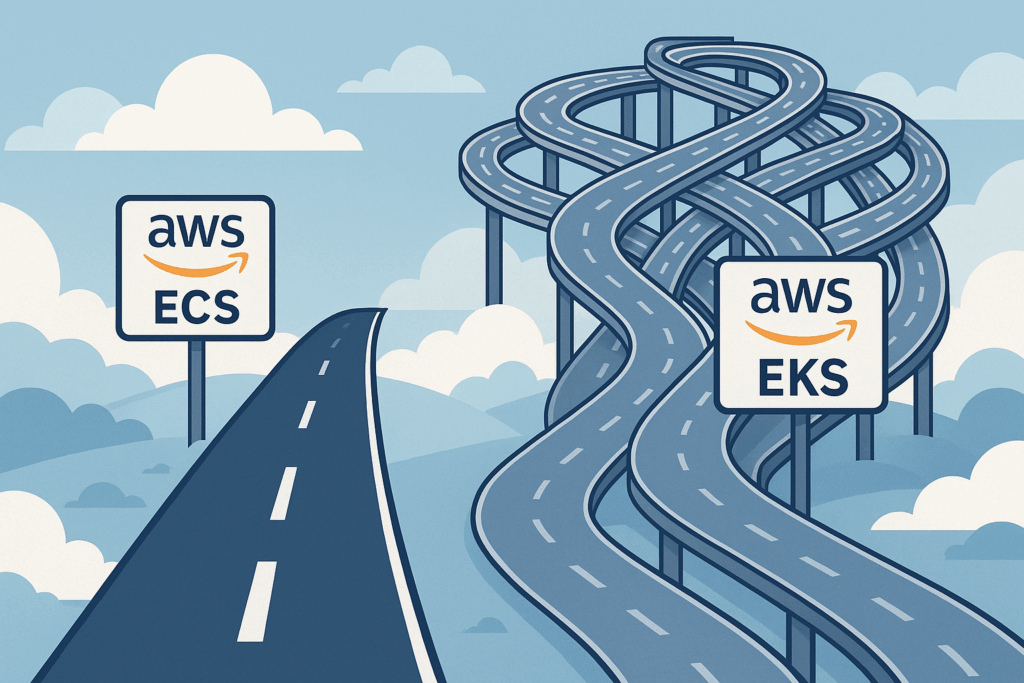
Selecting the right tools often feels like navigating a crossroads. Consider planning a significant project, like building a custom home workshop. You could opt for a complex setup with specialized, industrial-grade machinery (powerful, flexible, demanding maintenance and expertise). Or, you might choose high-quality, standard power tools that handle 90% of your needs reliably and with far less fuss. Development teams deploying containers on AWS face a similar decision. The powerful, industry-standard Kubernetes via Elastic Kubernetes Service (EKS) beckons, but is it always the necessary path? Often, the streamlined native solution, Elastic Container Service (ECS) paired with its serverless Fargate launch type, offers a smarter, more efficient route.
AWS presents these two primary highways for container orchestration. EKS delivers managed Kubernetes, bringing its vast ecosystem and flexibility. It frequently dominates discussions and is hailed in the DevOps world. But then there’s ECS, AWS’s own mature and deeply integrated orchestrator. This article explores the compelling scenarios where choosing the apparent simplicity of ECS, particularly with Fargate, isn’t just easier; it’s strategically better.
Getting to know your AWS container tools
Before charting a course, let’s clarify what each service offers.
ECS (Elastic Container Service): Think of ECS as the well-designed, built-in toolkit that comes standard with your AWS environment. It’s AWS’s native container orchestrator, designed for seamless integration. ECS offers two ways to run your containers:
- EC2 launch type: You manage the underlying EC2 virtual machine instances yourself. This gives you granular control over the instance type (perhaps you need specific GPUs or network configurations) but brings back the responsibility of patching, scaling, and managing those servers.
- Fargate launch type: This is the serverless approach. You define your container needs, and Fargate runs them without you ever touching, or even seeing, the underlying server infrastructure.
Fargate: This is where serverless container execution truly shines. It’s like setting your high-end camera to an intelligent ‘auto’ mode. You focus on the shot (your application), and the camera (Fargate) expertly handles the complex interplay of aperture, shutter speed, and ISO (server provisioning, scaling, patching). You simply run containers.
EKS (Elastic Kubernetes Service): EKS is AWS’s managed offering for the Kubernetes platform. It’s akin to installing a professional-grade, multi-component software suite onto your operating system. It provides immense power, conforms to the Kubernetes standard loved by many, and grants access to its sprawling ecosystem of tools and extensions. However, even with AWS managing the control plane’s availability, you still need to understand and configure Kubernetes concepts, manage worker nodes (unless using Fargate with EKS, which adds its own considerations), and handle integrations.
The power of keeping things simple with ECS Fargate
So, what makes this simpler path with ECS Fargate so appealing? Several key advantages stand out.
Reduced operational overhead: This is often the most significant win. Consider the sheer liberation Fargate offers: it completely removes the burden of managing the underlying servers. Forget patching operating systems at 2 AM or figuring out complex scaling policies for your EC2 fleet. It’s the difference between owning a car, with all its maintenance chores, oil changes, tire rotations, and unexpected repairs, and using a seamless rental or subscription service where the vehicle is just there when you need it, ready to drive. You focus purely on the journey (your application), not the engine maintenance (the infrastructure).
Faster learning curve and easier management: ECS generally presents a gentler learning curve than the multifaceted world of Kubernetes. For teams already comfortable within the AWS ecosystem, ECS concepts feel intuitive and familiar. Managing task definitions, services, and clusters in ECS is often more straightforward than navigating Kubernetes deployments, services, pods, and the YAML complexities involved. This translates to faster onboarding and less time spent wrestling with the orchestrator itself. Furthermore, EKS carries an hourly cost for its control plane (though free tiers exist), an expense absent in the standard ECS setup.
Seamless AWS integration: ECS was born within AWS, and it shows. Its integration with other AWS services is typically tighter and simpler to configure than with EKS. Assigning IAM roles directly to ECS tasks for granular permissions, for instance, is remarkably straightforward compared to setting up Kubernetes Service Accounts and configuring IAM Roles for Service Accounts (IRSA) with an OIDC provider in EKS. Connecting to Application Load Balancers, registering targets, and pushing logs and metrics to CloudWatch often requires less configuration boilerplate with ECS/Fargate. It’s like your home’s electrical system being designed for standard plugs, appliances just work without needing special adapters or wiring.
True serverless container experience (Fargate): With Fargate, you pay for the vCPU and memory resources your containerized application requests, consumed only while it’s running. You aren’t paying for idle virtual machines waiting for work. This model is incredibly cost-effective for applications with variable loads, APIs that scale on demand, or batch jobs that run periodically.
Finding your route when ECS Fargate is the best fit
Knowing these advantages, let’s pinpoint the specific road signs indicating ECS/Fargate is the right direction for your team and application.
Teams prioritizing simplicity and velocity: If your primary goal is to ship features quickly and minimize the time spent on infrastructure management, ECS/Fargate is a strong contender. It allows developers to focus more on code and less on orchestration intricacies. It’s like choosing a reliable microwave and stove for everyday cooking; they get the job done efficiently without the complexity of a commercial kitchen setup.
Standard microservices or web applications: Many common workloads, like stateless web applications, APIs, or backend microservices, don’t require the advanced orchestration features or the specific tooling found only in the Kubernetes ecosystem. For these, ECS/Fargate provides robust, scalable, and reliable hosting without unnecessary complexity.
Deep reliance on the AWS ecosystem: If your application heavily leverages other AWS services (like DynamoDB, SQS, Lambda, RDS) and multi-cloud portability isn’t an immediate strategic requirement, ECS/Fargate’s native integration offers tangible benefits in ease of use and configuration.
Serverless-First architectures: For teams embracing a serverless mindset for event-driven processing, data pipelines, or API backends, Fargate fits perfectly. Its pay-per-use model and elimination of server management align directly with serverless principles.
Operational cost sensitivity: When evaluating the total cost of ownership, factor in the human effort. The reduced operational burden of ECS/Fargate can lead to significant savings in staff time and effort, potentially outweighing any differences in direct compute costs or the EKS control plane fee.
Acknowledging the alternative when EKS remains the champion
Of course, EKS exists for good reasons, and it remains the superior choice in certain contexts. Let’s be clear about when you need that powerful, customizable machinery.
Need for Kubernetes Standard/API: If your team requires the full Kubernetes API, needs specific Custom Resource Definitions (CRDs), operators, or advanced scheduling capabilities inherent to Kubernetes, EKS is the way to go.
Leveraging the vast Kubernetes ecosystem: Planning to use popular Kubernetes-native tools like Helm for packaging, Argo CD for GitOps, Istio or Linkerd for a service mesh, or specific monitoring agents designed for Kubernetes? EKS provides the standard platform these tools expect.
Existing Kubernetes expertise or workloads: If your team is already proficient in Kubernetes or you’re migrating existing Kubernetes applications to AWS, sticking with EKS leverages that investment and knowledge, ensuring consistency.
Hybrid or Multi-Cloud strategy: When running workloads across different cloud providers or in hybrid on-premises/cloud environments, Kubernetes (and thus EKS on AWS) provides a consistent orchestration layer, crucial for portability and operational uniformity.
Highly complex orchestration needs: For applications demanding intricate network policies (e.g., using Calico), complex stateful set management, or very specific affinity/anti-affinity rules that might be more mature or flexible in Kubernetes, EKS offers greater depth.
Think of EKS as that specialized, heavy-duty truck. It’s indispensable when you need to haul unique, heavy loads (complex apps), attach specialized equipment (ecosystem tools), modify the engine extensively (custom controllers), or drive consistently across varied terrains (multi-cloud).
Choosing your lane ECS Fargate or EKS
The key insight here isn’t about crowning one service as universally “better.” It’s about recognizing that the AWS container landscape offers different tools meticulously designed for different journeys. ECS with Fargate stands as a powerful, mature, and often much simpler alternative, decisively challenging the notion that Kubernetes via EKS should be the default starting point for every containerized application on AWS.
Before committing, honestly assess your application’s real complexity, your team’s operational capacity, and existing expertise, your reliance on the broader AWS vs. Kubernetes ecosystems, and your strategic goals regarding portability. It’s like packing for a trip: you wouldn’t haul mountaineering equipment for a relaxing beach holiday. Choose the toolset that minimizes friction, maximizes your team’s velocity, and keeps your journey smooth. Choose wisely.